- Zhwan Mohammed Khalid
- [email protected]
- 0750 420 3929
- Zhwan Mohammed-72860064
-
Urine microscopic examination is critical for the diagnosis and monitoring of
individuals suspected of having renal disorders or urinary tract infections. Traditionally, urine sediment smears are examined under a microscope for manual counting
and categorization of urine sediments. This makes a time-consuming and laborintensive process. Also it is susceptible to human mistakes and the skill of the human
observer, and cannot satisfy today's clinic requirements. Clinicians and patients
would benefit greatly from an automated method that can analyze and quantify urine
sample images.
In this study a deep learning method is proposed for analyzing urinary particles. In the first step microscopic images of urine sediment ware collected in real
human urine, which combined 820 images for four cell classes: Red Blood Cell
(RBC), Calcium Oxalate, Cysten, and Uric acid. Then some preprocess on images to
be specified and clearer are provided to get a good result. In the next step for classification and analyzing urine sediment two methods are presented. In the first method
after making preprocess the image directly goes to classification algorithms, for this
method Convolution Neural Network (CNN) structure and five ConvNet models
such as MobileNet, VGG16, DenseNet, ResNet50V, and InceptionV3 have been
proposed. Therefore, in the second method before images go to classification algorithms feature extraction is performed. 22 features from the images are extracted,
and then numeric dataset is prepared and normalized it. Lastly, the normalized dataset have been introduced to the classifiers to distinguish subjects. For that purpose
two different classifiers have been employed including Support Vector Machine
(SVM) and K-Nearest Neighbor (KNN). After performing these two ways by using
eight classification models and Training on the real dataset, it was realized that the
best model is MobileNet. This model achieved the highest accuracy of 98.3%. Also,
InceptionV3 and DenceNet have comparable accuracy results with 97.5%. And the
proposed CNN structure has a good result with 96.12%. This study will be greatly
beneficial for clinicians to automate, analyze, and quantify urine sediment images. - Erbil Technical Engineering College
- Information Systems Engineering Department
- Machine Learning
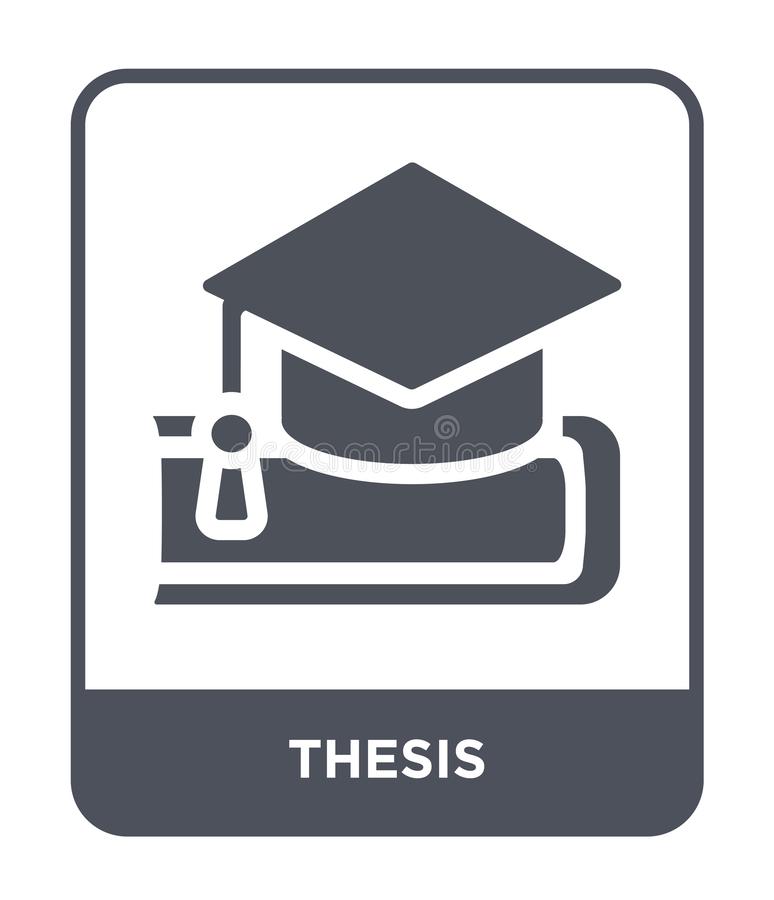