- Zrar Khald Abdul
- [email protected]
- 0773 064 8822
- Dissertation_Zrar Khald Abdul_toprint
-
Abstract:
The automatic fault detection in rotating machinery has emerged as crucial factors for ensuring the high reliability of modern industrial systems. Therefore, developing automatic fault detection is a vital challenge in modern industry. This dissertation intends to develop an automatic model based on machine learning to detect gear faults. During the development of an automatic model for gear fault detection, certain issues are found that need to be addressed to establish a reliable monitoring system. Firstly, the literature has not yet explored the potential of representing vibration signals, despite their time-series nature, using both non-time-series based and/or time-series-based features. Secondly, the vibration signal may have different channels based on the type of accelerometer sensor. A lack of studies is noticed to show the impact of the representation of these channels on the performance of the fault detection system. And thirdly, the fault diagnosis process for rotating machinery becomes challenging due to the non-stationary and non-linear characteristics that commonly arise from varying operating conditions. Various conditions make it difficult for traditional linear to effectively capture the underlying fault patterns.
To address these problems, some investigations are required to be studied including investigating various feature representations for fault detection, investigating the nature of fault detection in terms of time series or non-time series analysis, investigating various forms of fusion models using traditional concatenation or adopting multi reservoir to model with multi-channels, and investigating time-consuming of model.
Regarding the utilized features, Mel Frequency Cepstral Coefficients (MFCCs) and Gamma tone Cepstral Coefficients (GTCC) have been adopted as a feature and various forms of these features have been used to feed two main different models including the time series model and non-time series model. For the time series model, Long Short-Term Memory (LSTM) and Echo State Network (ESN) have been adopted to classify the gear faults. The high performance of LSTM is achieved for gear fault classifications despite its being time-consuming during the training phase. To avoid time consumption, the ESN, which consumes less time as some of their layer’s weight values are non-trainable and selected randomly. Further investigation has been studied by adopting multi channels reservoir which has led to achieving reliable gear fault detection.
Regarding the non-time series model, Support Vector Machine is fed by two different forms of the feature representation, the first of which is the statistical form called in this dissertation (stat-SVM). The problem with the statistical form is that it may lead to loss of some important features related to gear fault and it may lead to degradation in gear fault detection. To address this problem, we use a concatenation of the frames of both features (MFCC and GTCC) to be fed to the SVM (concat-SVM). Consequently, a high-performance rate of gear fault detection is achieved. Further, investigation is adopted by optimizing the features hyperparameters as both features were originally designed to extract features from speech signals. For this purpose, Grey Wolf Optimization (GWO) and Fitness Dependent Optimizer (FDO) have been utilized to optimize three hyperparameters of both GTCC and MFCC. The performance of optimizing the hyperparameters of MFCC has not shown any improvements. Oppositely, improvement in GTCC performance by the same optimization process is observed and validated.
All the proposed models have been evaluated by two public datasets namely, Prognostic Health Monitoring 2009 (PHM09) and Drivetrain Dynamic Simulator (DDS). Based on the result, non-time series model demonstrated superior performance compared to time series models within a margin of 2 to 15% accuracy.
- Erbil Technical Engineering College
- information system engineering
- Gear fault detection using machine learning algorithm.
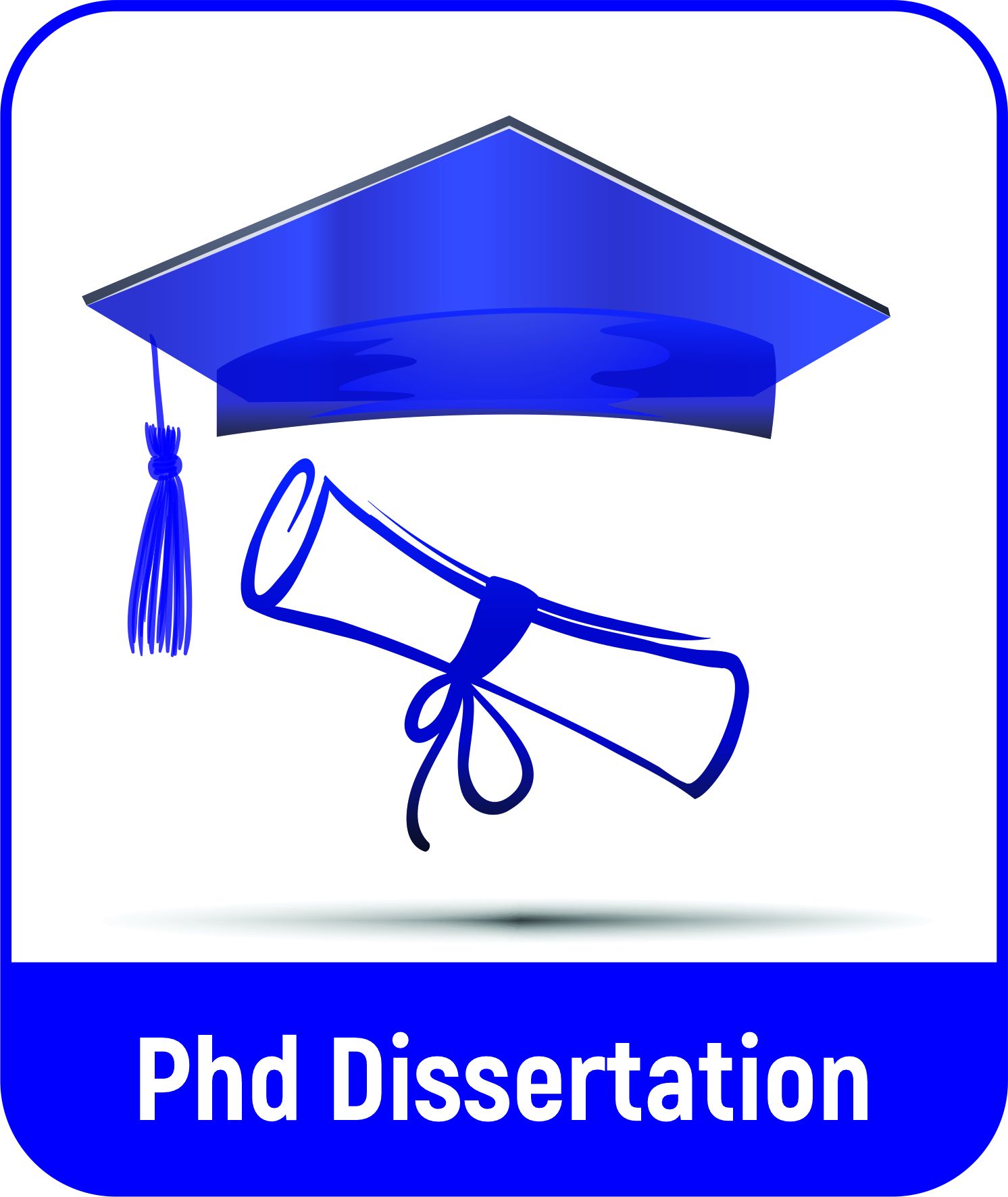