- Chiman Haydar Salh
- chiman.salh@epu.edu.iq
- 0750 479 4161
- Disertation new1
-
Breast Cancer (BC) is a prevalent and potentially life-threatening disease affecting women worldwide. The timely and precise identification of ailments is essential in enhancing patient outcomes and rates of survival. Deep learning models have emerged as powerful tools for medical image analysis that potentially aiding in automatic BC detection. Several studies have been done in this area, and many gaps should be considered in the survey. As there are no datasets related to the Kurdistan Region of Iraq (KRI) hospitals categorizing images based on breast cancer cases, much of the writing on this topic has focused on classification accuracy without addressing reliability until now. This dissertation intends to develop a model based on machine learning that can be used to detect BC.
In order to be able to swiftly and cost-effectively identify potential cases of breast cancer. In this dissertation, very robust approaches for detecting breast cancer, particularly mastectomy and Wide Local Excision (WLE) were developed. BC datasets have been constructed, including magnetic resonance imaging (MRI) (DCE-MRI) stands for dynamic contrast-enhanced magnetic resonance imaging and mammography.
This dissertation intends to develop a model based on deep learning that can be used to detect breast cancer. It presents three main methods which can be categorized as follows:
This novel approach aims to classify breast cancer MRI images through a three-stage process: segmentation and feature extraction using five techniques it is (Scale-Invariant Feature Transform (Sift), Histogram of Oriented Gradients (HOG), Edge-Oriented Histogram (EOH), Local Binary Patterns (LBP), and Bag of Words (BoW) ), and classification using six algorithms it is (K-Nearest Neighbors (KNN), Artificial Neural Network (ANN), Support Vector Machine
(SVM), AdaBoost, Decision Tree (DT), and Random Forest(RF) ). The method demonstrated promising results, with 91.9% accuracy for images from Rizgary Hospital - Erbil and Hiwa Hospital - Sulaymaniyah, 97% accuracy on the ACRIN dataset, and 92.3% accuracy for breast cancer MRI images, highlighting its effectiveness in BC diagnosis via MRI imaging.
The second models, which are convolutional neural network (CNN), ResNet152V2, and Mask Region-based Convolutional Neural Network (Mask R-CNN), have been used to develop a cancer mammography image classification and recognition model from authentic images with less training time and computation cost but high accuracy and recall which would be the target of the experiments. It has been concluded that ResNet152V2 achieved a higher accuracy of 100% in recognition of the type of breast density and normal or abnormal images. A modified CNN has been used to determine whether the mammogram image is left or right. This model used Mask RCNN to differentiate between malignant and benign tumors and find the tumor size.
The third model uses different deep-learning approaches to increase the deeper features in breast cancer MRI and DCE_MRI images. This model has EfficientNetV2L, Mask R-CNN, Detectron2, and Detectron2 with Faster RCNN. In this model, we used Yolov7 instead of Mask RCNN. Has been concluded that Mask R-CNN achieved higher accuracy in recognition by more than 10% than YoloV7. This dissertation has been extended to the automatic detection of breast cancer for mastectomy or WLE using different deep-learning models.
In conclusion, incorporating deep learning models into breast cancer diagnostics yields promising outcomes in accuracy and efficiency. These models can potentially be helpful tools for radiologists and pathologists in detecting and classifying breast cancer.
- Erbil Technical Engineering College
- Information Systems Engineering
- Machine Learning
Dr. Shara Kamal, a lecturer at Erbil College of Technology of our university participated in the United Nations Climate Change Conference -2023 in Dubai, UAE.
Dr. Shara Kamal and the Kurdistan Regional Government delegation On the sidelines of the conference, he participated in a workshop on green energy investment in the Kurdistan Region in Dubai, UAE.
Members of the Kurdistan Regional Government delegation presented their reports and opinions on the issue.
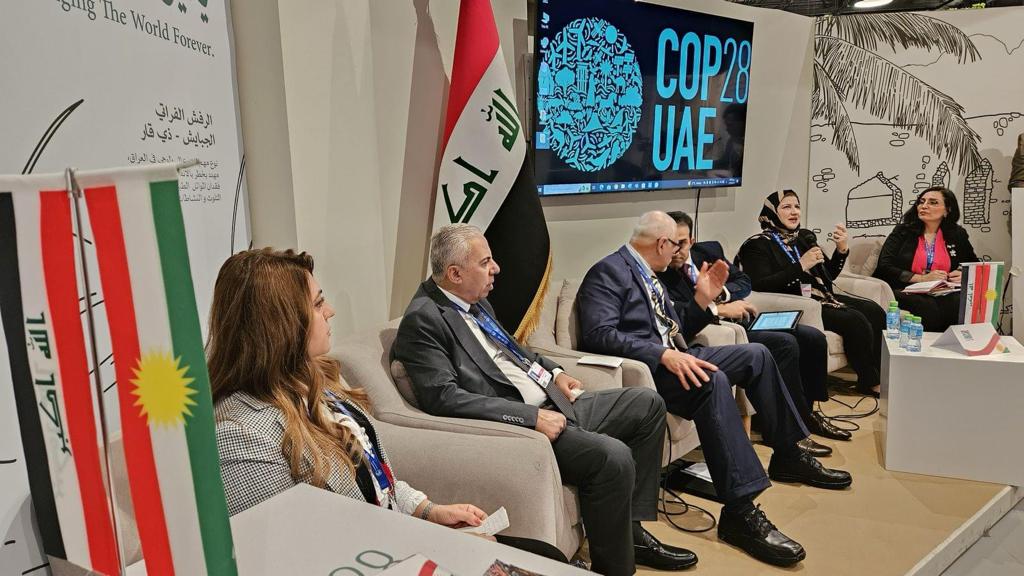
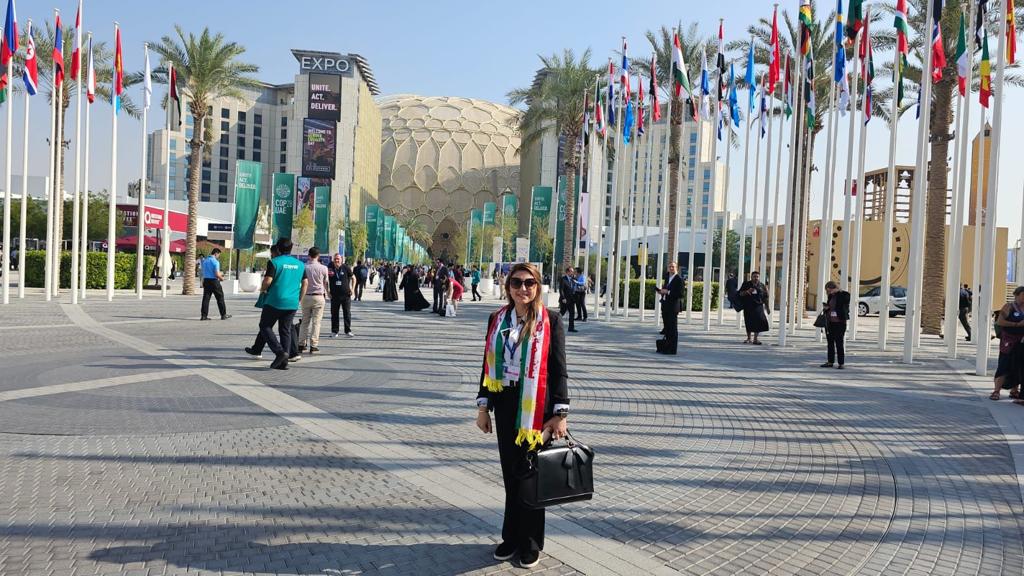
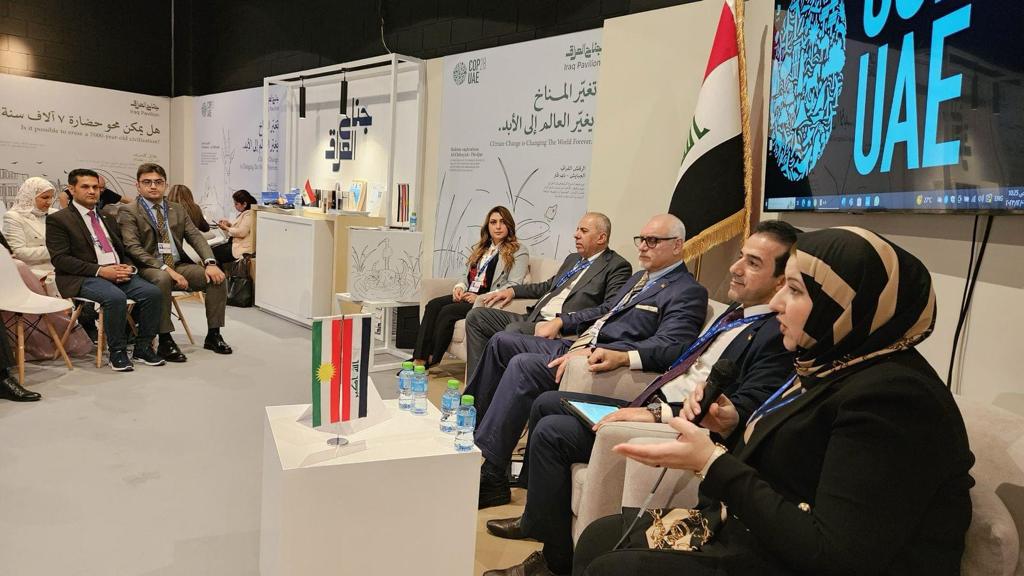
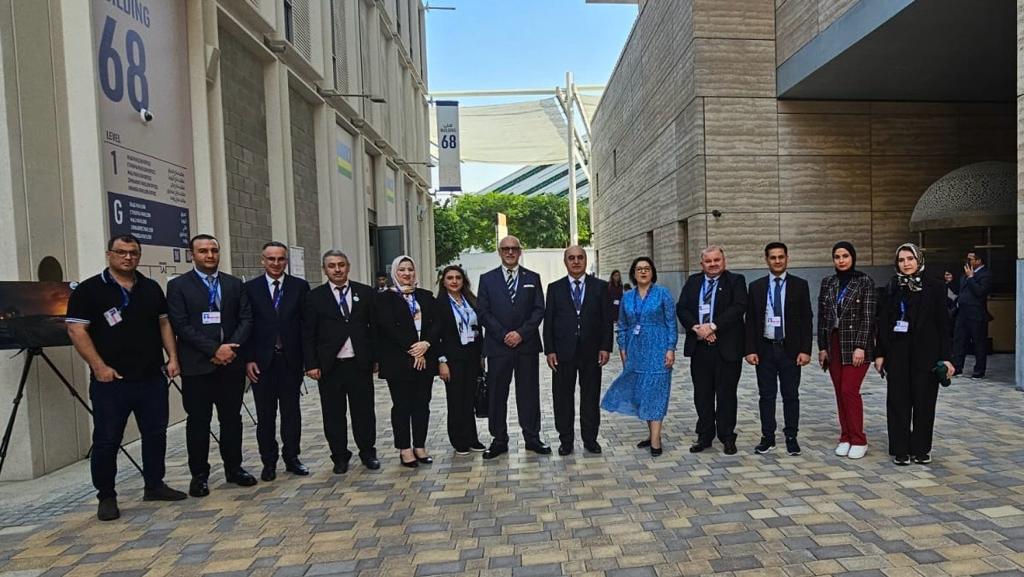
In the name of the Allah
Dear Presidency of Soran University, teachers, colleagues, students and families of the victims.
Sadly, the burning of a building was an unexpected and tragic disaster that caused heartbreaking victims.
We extend our deepest condolences to the families of the victims. We hope that God will heal the injured, forgive the deceased and bless them with His Paradise.
M. Soran Thanoon Yasin Hamra
Dean of Khabat Technical Institute – Erbil
Erbil Polytechnic University
On Wednesday, the 6th of December, 2023, Asst. Prof. Nageb Toma Bato, the Vice Rector of the Erbil Polytechnic University for Scientific Affairs participated in the second meeting of the High Steering Committee of ESDEHARAK Project.
The meeting aimed to evaluate the work and activities of the project during the past six months and to plan activities for the next six months.
This project focuses on training and upgrading the skills of university staff for employment and agricultural development centers. It is implemented by Cowater in collaboration with the Ministries of Higher Education, Scientific Research, Education, Agriculture and Social Affairs of the Kurdistan Regional Government and the Federal Government.
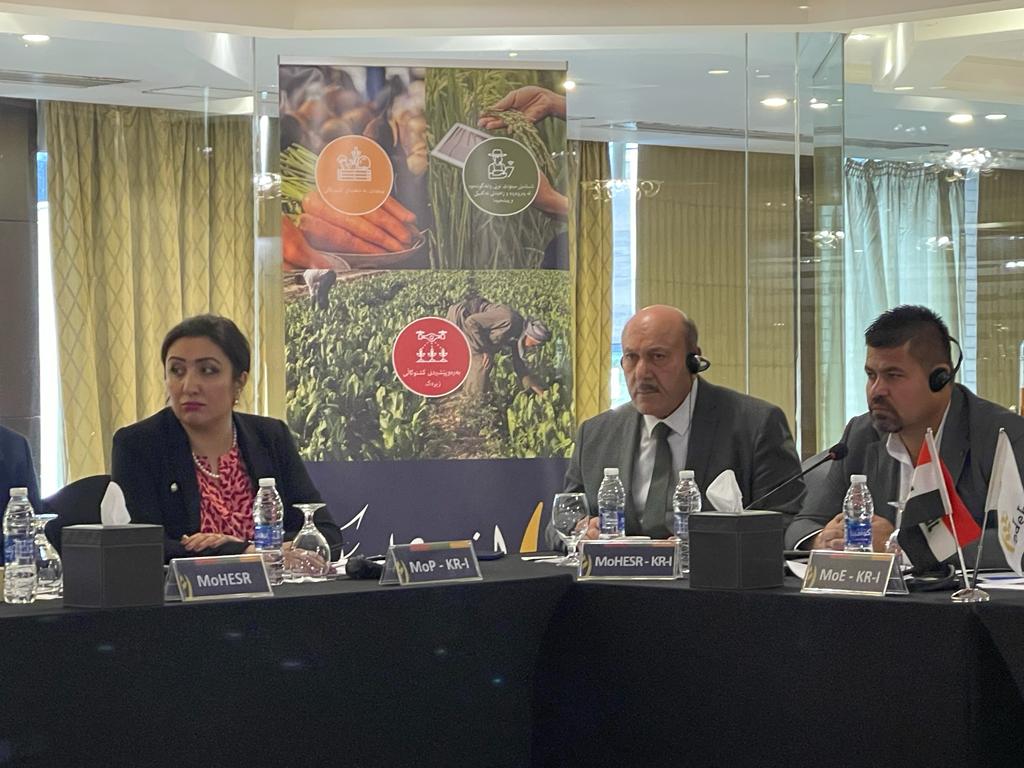
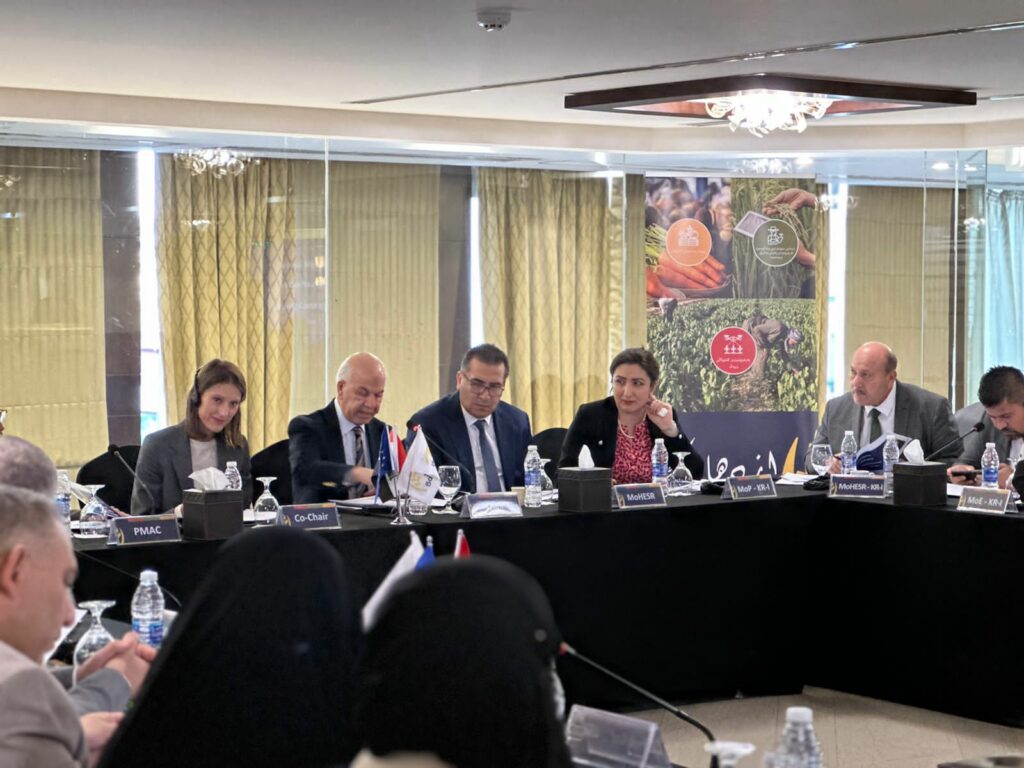